Industrial Data Labs on Oil and Gas Startups
0:00 This podcast is brought to you by EnergyX. Are you tired of paying huge rates to the big cloud providers? Are you worried about being booted off a cloud platform? If your company doesn't meet their
0:10 ever shifting standards, ready to step up your data security and disaster recovery game? Well, ladies and gentlemen, your new cloud is ready. Introducing XCloud, the scalable, resilient,
0:19 computing cloud that is also actually affordable. It's high performance compute for half the cost. HPC for HTC XCloud from Red Team is opening a beta program for new cloud computing customers, and
0:32 that means you, my friend. This cloud is powered by the XMDC, immersion-cooled modular data center from EnergyX. I've seen the data center operation and it is a total game changer. So if you want
0:42 more information about the beta launch, go to the URL in the description. Type in promo code betaBETA for 50 off of your first instance. And so the URL is going to be digitalwalkcutterscomenergyx.
0:57 What's up, Walkhead? Welcome back to UNF, so we're going to start as a podcast. I'm here, it's not every day that I'm here with somebody that I've known for longer than most people in this space.
1:06 Marty and I go way back. I don't know, we've done each other since probably 2013, 2014. Yeah, I'm a be honest with you. I don't even remember how we met. Do you remember? Yeah, I think I do.
1:16 I think I cold called you. Really? I think so. I think you were doing a startup with GDSware. Yeah And at that time, I was with Signet Interactive, who no longer exists, doing, trying to do an
1:31 oil and gas division for our marketing services. Oh, yes. Yes. Yes. Yes. That's how long ago it was.
1:39 I remember. And I came and - Who was the guy who worked over there? Chris Mulgrew. Yes. Oh, no, there's a different guy, a different guy. Jeff James. Different guy. I don't know. Different
1:50 guy, you know him I know him because it was after I had left GDS where he'd offered me a job. Really? Yeah, he was like, Come work with Signet. It was one they were still going. And then we had
2:03 hosted our first event with Digital Walk Haters years later. I was like, Yeah, I'll put my name in for me, whatever.
2:08 And he was just like, Oh yeah, you're glad you didn't join. Things that really didn't work out well for us. And I was like, Oh.
2:15 I was like, All right, good. I think I know who you're talking about. Good to know. Rich. Rich, yes. Rich, that's who it was. Yeah, he's a great, great guy. I like him a lot. Yeah, Rich
2:24 is awesome So, for those of you who didn't make it to Energy Tech Night, okay, see, Marty was the winner. Yes. With Industrial Data Lab. Thank you for having us. What I love, what I love
2:34 about seeing, like you guys just close around to funding, you just want Energy Tech Night, you think you're getting a lot of publicity, a lot of investors are reaching out. And what I love about
2:43 this is that not many people know outside of like me, Aaron, your wife, how long you've been grinding to like solve a singular problem And I think that you have dove deeper into a sector that I've
2:59 seen any founder ever. And you have picked apart this thing, you have got inside their minds and understood exactly what their challenges are. And you're like, I'm going to crack this. And like
3:09 you guys have finally, like you have cracked it in like the best kind of way. Yes. And it was so cool just to see, like I've seen, I saw the very first iterations back when you guys were doing
3:19 stuff, you know, like sell or Google sheets, however you're doing it, and then see what you guys had in your detect night And then for the crowd to also just to appreciate that at the same time
3:28 was just a really, really cool moment. So I'm excited to dive in for everybody who's listening here. What is at high level like what do you guys do? So industrial data labs were an applied
3:38 artificial intelligence company and we're solving the manual TDS,
3:44 highly technical sales and sourcing inefficiency problem within industrial markets. And so how we do that, is we're building proprietary artificial intelligence. And our main objective is how do we
3:59 surface this AI
4:02 to gain the most value for our customers? And then we're surfacing it inside of workflows. So existing workflows. So I think if I understand it correctly, when you're in, you guys are servicing
4:14 particularly just the valve market right now, right? So pipes, valves, fittings, and flanges. Okay, so all customers. Yep, and distribution and manufacturers Okay, our customers. And so if
4:24 somebody is ordering, or they're trying to put it in order to either distributor or to a manufacturer, the way that I understand it is, they send in either an email or a spreadsheet attached to an
4:35 email with like 20, 000 lines of technical specifications of what they're looking for, right? And then the way that it's done currently is somebody will take that spreadsheet and then manually go
4:48 through, and I'm guessing they're checking their system, if it's available, they're checking to see if. probably a bunch of other things. How long is that, is that days, is it weeks, is it?
4:59 So it depends. So there's really two use cases that we're solving for. So one is gonna be the bill of material use case which you described. So hey, we wanna build this project. The project is
5:10 three years away or we need this quote finalized in six months. A CAD system literally will output a spreadsheet and it will list 10, 000 pipes, thousands of functions And each of the descriptions,
5:24 so if you figure what is a valve description or a pipe description. So it's essentially a series of attributes defining what something is. So for a valve, the attributes can be a type size,
5:35 pressure class, all the way to body material, trim material, the end connection, the operator. So it was a hand wheel,
5:45 maybe ID, OD, all that kind of stuff. All the specifications, even supplemental, with type of paint, right? And you have 10, 000 lines dealing with that technical information and you're
5:60 receiving it in a spreadsheet. So pretend you're in an inside sales rep. I'm gonna email you this spreadsheet with 10, 000 lines with all those attributes associated with it. Now, if you're kind
6:13 of a part of one of these distributors and manufacturers today, you're
6:18 really involved in this industry in two ways Either you're fairly new or you're very experienced. If you're experienced, you can get through those maybe in eight hours, 10 hours. But the first
6:28 step in really solving this large bill of material problem is these valve attributes or these equipment attributes live in one cell of a spreadsheet, right? So it's almost impossible to sort through
6:42 that spreadsheet unless you go line item by line them in your ERP. So what people do is these inside sales rep depending upon the inside sales team or the rep particularly. they've created templates.
6:54 And so they'll take a spreadsheet, right? You'll take a spreadsheet for me. If I'm Shell, I send it to you. And you take all of the data in that spreadsheet, you organize it in another
7:04 spreadsheet, right? So that can take a full day. Sometimes it takes three days just to organize one project, one spreadsheet. And then your next step is to Google search every line item. Inside
7:16 of the Google search, literally go in your ERP, go into where you can search for items and you're typing in every item. Unless you've memorized every product code for every item, right? That
7:28 takes forever. So what the problem is today is there's distributors. So we have a customer who's only getting to 50 of these large bill materials coming in. And you can imagine only getting to 50
7:39 of 5, 10 million. Yeah, 50 of inbound business. And it's like, we just literally can't. Right. 10 to the rest of it. And these guys are tasked to grow 3X over the next five years. Well, how
7:49 do you do that? If you hire more inside sales reps, what happens? You got to train them. It takes a couple of years to train on technical data. Really? You steal from another company, possibly.
7:59 Also, who wants those jobs? Right?
8:04 I blow my brains out if I had to do that every day, let me be honest. I actually heard a story of one of our, one of the customers that we're fairly close with, we're having a hard time recruiting
8:14 for some of these positions.
8:18 And they had their recruiter take applications and go to Chick-fil-A and hand out applications through the drive-through to try to recruit these Chick-fil-A members because they're detailed organics
8:28 to get them in their inside sales team. But it's hard, it's hard to recruit. And then the interesting thing that we're finding is, so there's a lot of younger, ambitious people starting to become
8:41 management. VP is right and they're aggressive. They wanna make money. They wanna grow their particular company's market share and they're looking for all sorts of tech. technology to do that
8:52 because they know the one hole in their organization is increasing the size of their inside sales team and how do you do that effectively? How do you do that efficiently? It's very difficult for
9:04 them to do. And so you have ambition with a lot of obstacles to it. And so technology, you know, and what we've seen from our customers is kind of the answer to solve a lot of their problems and
9:16 get them to where they want to go So you guys are taking this super tedious and time consuming process that is a massive bottleneck for these companies. And then what do you, how are you applying
9:29 the technology and how are you doing it? So our main objective right now is surfacing the value of the AI, right? And so the other component to that is the requirement that we've set for our
9:43 company is to do that inside of existing workflows So you've got to do that inside of an email or inside of a spreadsheet. where you're not having your customers try to adopt a new platform, a new
9:56 box, which makes it terribly difficult. Go to where they're working, so. Right, so here's a challenge. So we're building proprietary artificial. So our AI is ours. We're building it from the
10:07 ground up. And it's very, very difficult, but one of the things that you need to do in order to build the type of AI that we are is we need to capture thousands of these build materials, right?
10:21 We cannot go and scrape the internet for these descriptions because they're not real world descriptions. So the AI would be off if we just scrape the internet. So we've worked with our customers to
10:32 gather thousands of these build materials. And well, here's the challenge. So each of these build materials, whether it's from Saudi Aramco or someone here in North America, is different. So
10:44 descriptions are different. The design is different And so how do you create a way to automate? the ability to capture not only the spreadsheet ingested, but then take the difference between the
10:58 descriptions that we need out of those spreadsheets at scale and stuff that's not relevant to the problem that we're solving, right? And so we were working on that for two months and we got, I
11:10 don't wanna say lucky, but we've been really fortunate in the progression of our AI to where our AI team is, we can talk about them, top 1 of 1 and we're really lucky to find them. But we're able
11:25 to essentially kind of use our existing model to train another model in order to ingest PDF spreadsheets, whatever and understand the difference between a description that's valuable and something
11:39 that's not, maybe a location of a plant or a specific address or other types of language that are contractual languages so we can
11:48 take off these spreadsheets. and do hundreds of thousands, if not millions of descriptions. And then we have a team of labelers and they sit and we do, we're doing a 10, 000 batch labeling
12:03 project right now. And they'll go through. And they'll use to train the AI. So they'll literally log into our called label studio. And we've got hundreds of thousands of descriptions. And then
12:16 they'll go, well, this is the size. This is the pressure And literally trains the AI just for this industry. Geez. And so going to where their workflow is, is what y'all do is that built into
12:28 plugins in
12:30 Gmail, Outlook, Excel, things like that? So it depends on the particular company. So
12:38 right now, if we use the Excel example, so depending upon the company and how far we make it with our IT team, is right now we can do an Excel add-in. where you can essentially just highlight the
12:50 descriptions, click a button, a sheet pops up, all the descriptions are organized for you, and then the AI will search your ERP or a data set to find the right product codes for that particular
13:02 description. Or we're doing it another way just to start off with some users where we weren't able to really work with their IT team so far is where you can drag and drop the Excel spreadsheet. The
13:15 spreadsheet comes up in a good user interface, do the same activity, highlight the cells, click a button and it's done. To really you guys have thought about it, no matter how you want to come
13:24 play with this data, you have some sort of solution there. We're working towards the right solution. So we've been really lucky with the customers that we have. We meet with them every week to go,
13:33 okay, what do you think of this? Use this for the next week and they say, Hey, this is good, this is bad. Then we'll use our team to iterate based off of their feedback just to try to figure out
13:42 the right solution for not only our customers, but for the industry.
13:48 Let's go more into, I have so many questions, but let's go more into like your background in terms of like solving this problem. Yes. Right, like, I mean, the best way to phrase it is like,
14:00 what have you learned? 'Cause I know you've learned a lot. And I know there's been a billion different iterations and pivots and
14:07 just as you kind of uncover new things and new learning that kind of guides the direction of the company. But what I love about that is that you have embodied resilience and like perseverance of like,
14:17 no, we're gonna, like, we know that there's a problem here. We know it's a massive opportunity. And I'm not gonna let the fact that we haven't cracked it yet just turn us from figuring it out.
14:27 One day this will be figured out. Yeah. And I'm really hoping it's up. So what have we learned as the question? Yeah, what have you learned from like the beginning to just the 10? It's just the
14:35 10 now.
14:39 So the one thing, the one hypothesis that I had that turned out not to be true was that
14:45 These industries are. here, kind of antiquated from the outside looking in, they appear that they don't want to adopt new technology. And I found out probably in the last couple of years that that
14:58 is absolutely not true. What I think is the problem is, is if you take a tech startup and you start either on the west coast or the east coast, you're going to have a lot of opportunity in order to
15:09 secure capital to really grow your startup and solve whatever problem or use case you think you are going to solve initially right until you pivot and something else. But what I think is true is a
15:21 lot of startups from the coasts don't spend a lot of time in these industries who need their technology in order to truly understand every element of the use case so that they can build the proper
15:36 technology to solve the problem. I think what happens is people from the coast will come in with a technology, pitch the These guys will give it a shot. And then they'll go either it doesn't work
15:49 out and when we get into the details of why but they have a bad taste of their mouth from technologists on the coast coming in and trying to transform their company and bend a lot of money and it
16:01 doesn't work And these guys want technology and they want to solve a lot of these problems that I just don't think people are willing to do it it's not to say that somebody can't come in and crack.
16:14 The code and land upon something but you're definitely the outlier and I've seen that countless times of people who come in they build a widget They think it's applicable oil and gas they really don't
16:25 spend a time and time to dig deep and understand that the use cases right and they're just like well we've done this in X we've done this in X like we can come in and do the same thing oil and gas and.
16:35 For a variety of reasons some may be technical some of you like understanding some could just be cultural the fact they just don't know if she works. I'm not on their face and then they. back to
16:45 kind of whatever they were doing. The same is also true of, you can say, you know, kind of broader entrepreneurs in like the Q scenario and a lot of them are, you know, in oil and gas or energy.
16:56 And that persona is different, it's different than the coast. You know, you're dealing with people who are typically have spent a decade in, like their industry, this particular oil and gas and
17:08 then they go out and they identify a problem, they scratch their own itch and then they, you know, go and build something. Whereas the coast is, you know, you people are building stuff right out
17:16 of school and you may not have any sort of industry. They start a startup and then look for a problem to solve, right? Sometimes. Yeah, exactly. So, no, I've seen the same thing. I think some
17:26 of the best companies that have ever come out of this space have been built with people who've come from the industry. I agree. I think there's, there's such a deeper knowledge in terms of where
17:36 technology is applicable and truly solves a problem, right? Yeah, even even in solving this problem. So. You know, I go through, I think, on the presentation at the Tech Night in Oklahoma City.
17:51 So when people are trying to solve this problem for 25 years, it's the same thing every time. Let's build a platform, right? Let's disrupt distribution. And what we'll do is we'll work with the
18:02 end user and we'll come up with this platform and we can scale the platform through the end users. Then what we'll do is we'll onboard their suppliers, right? Well, how many suppliers do you think
18:10 an end user has? I've seen that so many times, by the way Tens of thousands, right? And then the way we'll onboard suppliers at scale is we'll get their contact information, we'll get maybe some
18:20 of their data, and we'll send them an email, hey, Shell's looking to buy from this platform, right? So that email goes into spam, likely, right? So no one sees it. Or a lot of these
18:30 manufacturers and distributors have a small technical team. So in a small sector, do you think they're gonna go and build out their data in order to fit your platform of which no one's buying
18:41 anything at all. And so you'll get that. first kind of bit of traction you think from the end user, and then once you try to onboard these suppliers, it's not going to happen. Then you got
18:52 distribution in North America. They are not going to let you come in and take any piece of their pie. It's just not going to happen. These platforms, whether it be e-commerce or workflow platforms,
19:04 they'll get a lot of press. These West Coast, these Coast VCs will be interested in platform. They love platforms. Then just over the course of time, just don't work out And I think what's
19:15 happening is companies want to disrupt the market. This market is not going to be disrupted. You need to build technology that provides value for each segment of the market in each customer within
19:29 that market, and you're not going to get anywhere. It's not going to happen. And you flip that, didn't you? Then you go to the manufacturer's master distributors and distributors and say, Hey,
19:37 we want to solve this problem, and started with them first. Yes. So we took a contrarian position. Most people start at the end user and go, hey, look, these are the people with the most money.
19:49 If we can create some sort of opportunity for suppliers to also make money with, we can kind of be that central figure in these transactions. And we said, you know, there's 25 years of a large
20:01 startup graveyard without a temp, let's go the opposite way. And so we went after distributors and manufacturers and said, hey, what's your problem? And we literally, I remember getting in the
20:13 car and we would drive around town and I would knock on doors and say, hey, look, we're trying to solve this problem. What do you think of this problem? And some people wouldn't invite us in and
20:21 talk about it. And we'd said, we said to a couple of manufacturers, what do you think about disrupting distribution? And they're like, never, we will never do it. We will never go outside of
20:30 distribution. And then something just clicked and we're like, okay, we're not gonna operate with any of the end users. Let's just stick distribution and manufacturing. And then from a sales side,
20:40 I mean, I love all the salespeople in this industry. and I love being able to help them solve their problems. And their problems are growth. So I remember when you first were telling me about this
20:54 years ago and you laid out the landscape of master distributors, distributors, but also like the manufacturing and just like how that ecosystem, like how it all kind of works. And it was a little
21:06 eye opening for me because I had never been exposed to that type of the industry. So let's just take a second to kind of give a quick little master class and like how all that works. On how kind of
21:18 this whole sourcing, yeah. So, but we'll go through the entire, let's do a little material problem, or even MRO kind of use cases. I need seven valves versus seven thousand. So Shell needs
21:30 valves, right? They go, okay, who do we buy valves from? And they'll send out a bill of material or an RFQ to their distribution partners. So sometimes they'll have multi-year agreements. with
21:42 certain companies, so they'll only use those companies as distributors. Right, yeah. And then other times they'll use whoever, whatever distributor kind of fits their needs at that point, or
21:51 they'll send out four or five emails to distribution teams. What happens is, you know, the distribution team and inside sales rep will get that email and they'll look to see if they have their
22:02 particular products in inventory or not. If they do not have an inventory, then they need to decide, well, who do I think has an inventory? That could be another distributor, so co-opetition,
22:15 or a master distributor. So a master distributor is often carry the things that distributors don't want to carry. For instances where, hey, I don't have this, I need to rely on these master
22:26 distributors in order to get this particular valve right away. Do they hold just rare inventory or like large quantities of inventory? So they'll do large quantities, but it's large quantities of
22:37 inventory that doesn't turn over often and they'll only sell two distributors. Okay, and then oftentimes maybe a main Asher distributor can't fulfill that needs and so the distributor will send it
22:49 to the manufacturer And then the manufacturers inside sales team will go do we have it? Do we not and sometimes they have it and they don't want to sell it to them because the manufacturers are
22:58 interested in selling 500 valves or 50 000, valves not one. Yeah, and so one of our customers kind of the use case and how we got inside of existing workflows, you know, he was a gentleman that
23:11 started using robotics in order to manufacture valves. And he was like, okay, this industry does a really great job at using machines in order to manufacture valves. So our back office or
23:24 operations are set and we've tried to get them as automated as possible, right? Well, as time went on, the front office grew and it became more and more manual, right? And it still exists today,
23:38 manual, people writing things down, taking a photo and texting it. happens.
23:44 And so now the objective is, well, we've got the manufacturing process automated. How can we automate the front office? Because I've got to compete with China. I've got to figure out how to get
23:56 leaner and leaner. And if I'm dealing with an end user who reduced my prices 10 years ago, well, my prices aren't going up. So where my margin needs to be had is on that front office space, right?
24:09 And so he was saying, you know, Marty, I'm getting these emails from these distributors for five valves. I don't want to sell five valves. And oh, by the way, the cost per quote for me is 300.
24:21 Well, my valves are 10. So if I sell five valves, I can sell 50 worth of valves, but it's costing me 300 top with this quote. We got to figure this out And so it's kind of an interesting dynamic
24:35 through the industry, I think, with this whole kind of sourcing sales process.
24:42 It's just, it's so wild, it's so wild that it's,
24:48 yeah. I mean, you're spot on in terms of everybody has tried to come in, build, go into the end users, build a software, get them on board. I've seen it so many times. And a lot of times it
24:58 kind of, their push is like, oh, we're just gonna build the Amazon for kind of boiling gas, right? Yep. And I think that's proven extremely, extremely difficult, right? And I don't know if
25:10 any of those have ever really had any sort of success or any sort of market share, to be honest with you.
25:16 I don't think so. I know there's a prominent one that raised a bunch of capital that I think eventually turned themselves into an actual distributor, versus being kind of the Amazon for boiling gas.
25:28 I think there's some traction happening in the chemical space where you can kind of create this market flow.
25:36 Our belief is that that is not the proper way to solve it I think what's going to happen is inside the end. industrial spaces, if you kind of figure the evolution of how people buy and sell things
25:47 when, you know, what did Amazon start 25 years ago, 30 years ago? So you think they're going to adopt 30 year old technology? I don't think so, right? I think there's a good opportunity for a
25:59 lot of these markets to skip that evolution of let's create some sort of e-commerce platform, even for themselves and get right into AI. And then with AI understanding pieces of equipment and
26:13 understanding attributes, so not only what it means to be a valve, but also understanding a type of size, these different attributes of a piece of equipment. Now that allows for systems to talk to
26:25 systems, right? So if there's a shutdown or turnaround happening, well, the data that is needed to figure out what's needed for that turnaround to happen properly, it's going to live either with
26:41 sometimes it's within a PDF file, right? Someone's desk or a CAD system, right? But now with the ability of true artificial intelligence understanding these markets, this ERP can communicate with
26:54 other ERPs throughout the entire supply chain. And this entire supply chain, if it works together, can start making decisions in order to take the time to kind of change some of the pieces of
27:06 equipment during that downtime from a month, six weeks into two weeks. And now they can do other things. And so there's so much value, I think, within AI, if you can really build it for these
27:23 markets and understand the use cases of which you can surface that AI for.
27:29 I mentioned that I had lunch with a guy earlier this week, who was in the pipe industry, and he was ranting and raving and how awesome y'all's tech was. But he was kind of going on a tangent about
27:39 just how - Even today, he feels like the entire RFQ, RFP process is just like wildly antiquated, right? He's in a different business now. And so with his startup, with them being evaluated in
27:53 the running for things, they're like, well, we have to put an RFP for two other companies. And he's like, there's no other company that does what we do. And they're like, well, we can't move
27:59 forward unless we have two more. And it's like, well, we're the only ones that do what we do. Do you feel like there's any other challenges, whether it be culturally, or would we tend logically
28:08 on possibly the EMP side that needs to change? Where they could adopt some of this technology. I think so. I mean, one of the challenges, or some of the challenges that we've been through is kind
28:22 of the data challenge. And data challenges is in two ways. So one, there's a lot of unstructured data that is very disorganized. It doesn't make a lot of sense living in a lot of these ERPs,
28:35 right? So even, you know, this microphone, let's say this microphone lived in my ERP. Well, it would leave my ERP as a black microphone or a microphone with a top on it or a microphone that you
28:46 could have. But it's the same thing, described three different ways inside of a ERP. So I know there's been a lot of money spent by venture capital trying to solve just that data problem.
28:56 But there's also the data privacy problem.
29:02 So this is my data. What are you going to do with it? And I don't want anybody seeing this data Well, in order to train AI, you need the data and you need private, confidential types of data.
29:16 And so I think that could be a cultural problem that may prevent a lot of opportunities or startups or even advancements to happen within these industries is
29:26 who's going to see the data? Am I open to giving it to a particular use case and have AI trained on it? So that could be an issue So like I mentioned, so, you know, I'm. When I'm not sitting
29:39 here podcasting, you know, spending a lot of my time talking with EPs. And even some of the big waffle service companies or technology companies about our new DWE Insight platform. And some of the
29:48 feedback that I've been getting as I'm talking to him like, hey, what are you guys looking at from technology perspective? What are your priorities, right? Trying to just kind of just keep my
29:55 finger on the poles of what's happening. And AI obviously keeps coming up in every single conversation. They're like, we're trying to wrap our heads around. Like we know there's an opportunity
30:04 here with AI. We're trying to wrap our head around the actual use cases of how can we apply this and the business. And with you guys building your own from the ground up, I think that that's
30:16 particularly interesting to a lot of these organizations because if you were to leverage a chat GPT, a cloud, a bard, it is something that your data is potentially gonna be used to train a much
30:28 larger model. You don't want confidential information, sensitive information to kind of get out there into the broader ether Right.
30:38 What advice would you have to those companies, even if it's some of these larger companies who are looking to start off with an AI, it's just called a project. Sure. Having built it from the
30:48 ground up, what advice do you have, what challenges, opportunities? Clearly define the use case. So we've defined specifically every single step that happens within this bill of material process.
31:01 From an inside sales rep, opening an email, reading the email, clicking the spreadsheet Spreadsheet opens up the description of how that spreadsheet can look, the data in the spreadsheet, the
31:13 value of different data aspects within that spreadsheet. And then we've had to go, so there's kind of the description of the use case problem, needs to be clear cut, fully described in detail,
31:27 right? Then you need the data, right? And so, you know, you can train or fine tune some open source smaller models to fit your company. and you have full control over that artificial
31:41 intelligence. But then you need to train the data. Well, how are you gonna do that? How are you gonna do it? So you can't hire people from overseas in order to train complicated technical data,
31:51 right? Especially when you're labeling it. So you need to find subject matter experts who will sit down. Within the company? Within the company. I would do within the company. I think there's a
32:02 large opportunity for people that maybe have retired and are looking for something to do and kind of give back to the community. And that's a lot of the gentlemen that we're using, retired PVF
32:13 experts, 40 years in the industry. And they give us a lot of advice, not only on our company, but they're helping us through this stabilizing labeling process. And the only way for the AI to
32:24 advance is to teach it what it needs to see. And in order to do that, you need the data and then you need the labeling in order to do that Do you think there should be any sort of
32:38 I don't know consortium you you've seen that time and time again, and I think that most of the time it ends up just being a People getting together and talking theoretical and nothing ever actually
32:48 like comes out of it But it's like I mean you see it with things like hugging face Yeah, you know where you've got just these super large open-source projects that we were contributing to it in
32:59 theory You would think that you can get a lot of people in oil and gas or an energy to kind of do the same thing and have your best people contributing to certain projects. Obviously, there's always
33:07 this fight over every time You have some JV's like that of like who actually owns it and you can use it and who could participate and all that kind of stuff Do you think it makes more sense people
33:17 just to do their projects internally and just you know fuck all the noise? Or do you think it makes sense for some of these companies to kind of come together or a third party to put something
33:24 together towards like Hey, we can build some cool open-source projects that's applicable to a lot of people in this space. I think it's going to be either But the best value these companies will get
33:35 is either to do it internally is going to cost portion. So the demand for AI is very high. The amount of people that can actually do it. Compute power is getting more expensive. Compute power is
33:46 expensive. We have our own GPU, believe it or not. Nice. Yeah. So, but the people that can do this, there's not that many of them. And here's another challenge. And kind of getting more
33:55 ingrained in the kind of the AI community or people that actually build this out. Well, the question that has been posed to us is not many of those people want to work in this industry.
34:10 Yeah. So now what happens? So these guys are making a million dollars a year at open AI, Google, Facebook, even if you were to pay them 3 million. So we we've kind of presented some situations
34:23 and we're like, we'll pay you double. No, I'm not I will not work inside of oil. I guess I was like, pay triple now. I was like, okay, so this is not about money. It's not about money.
34:36 building artificial. I want to build artificial intelligence. And I'm drawing a line in the sand over this moral conundrum that exists. What is the makeup of
34:46 this AI engineer? Is this typically like a software engineer or architect who's been doing this for a long time, who has now developed a particular skill set around building AI models or is it
34:59 something totally different? So for us it's going to be,
35:05 we call them kind of eccentric mathematicians. So people that understand different areas of mathematics and can apply it in these cutting edge ways to data in order to use not only the tools out
35:22 there, but also to build
35:26 our own kind of mathematics based off of what we're looking to achieve. So it's going to be a mixture of data scientists. Um, some people that are highly intelligent around the implications of AI,
35:42 how it can be used within different use cases. And then kind of the other team that we have is more of a user experience team. And those three elements fitting together is what makes this come alive.
35:57 Oh, man, there's so much, there's so much more stuff, but I know we're a little press on time. Unfortunately, um, dude, I love talking with this stuff And I just, I can't tell you enough how
36:07 excited I am to see the traction that you guys have. Yeah, thank you. Thank you guys for all your help. No, it was, it was just, it was just really cool to, uh, it was really cool to see
36:15 because I know how hard you've been working behind the scenes. But I think that this is a good lesson for other entrepreneurs that you want to solve a problem, truly understand the problems for the
36:26 people that you're trying to solve them for. Yeah. Right. And I think that you've, you've absolutely embodied that. So it's no surprise to me that it's, that it's working, you know, so, um,
36:35 In closing, so you guys raised some capital recently. What are the plans now in terms of growth? So we just brought on a VP of operations Awesome. Early two nights ago, we're hiring a full stack
36:46 engineer who understands some machine learning ops, but also has some good background in kind of user interfaces and how to really surface the value of the AI. For their build out our AI team and
36:59 then work with our customers to really make it come alive within their existing workflows and advance it from there. What's the, for those who are curious, what's the business model? How do you
37:11 guys monetize? We're actually, so we're trying to figure that out now. So there's one aspect where we can have a build a material, co-pilot, where our assessments, if you're an inside sales rep,
37:19 you
37:21 get this build a material and you can use our AI within your spreadsheet to work. So we have that more license-based model, but there's also a utility-based model that we're thinking of, to where
37:33 if you figure. You know, you're an end-user and I'm a distributor. We have a multi-year agreement. You keep sending me the same stuff. Well, we can plug our AI within the email system, can read,
37:44 understand what's being emailed. Therefore, I understand what you are wanting. I'm also connected to your ERP system, so I know what you have, right, and the price it's at. As a result, we can
37:55 capture email, do work on it, come up with an answer, import that into your ERP, send back to their system in a couple of seconds So we're trying to consider if a usage-based model works for us,
38:07 where we can just plug in the system, and then, depending upon how often you use DAI or you tap into it, you pay us some sort of amount of money. Yeah. But we're trying to figure that out. I
38:19 feel like there would also be an opportunity for a percentage of upside, like in your example of, the customer who turns down 50 of new business, like being able to quantify that and say, Hey,
38:28 we'll just take 10 of the upside that we were able to create for you. That's what we're working on that now, is so I think one of the next steps. So we like to sit behind our users' computers and
38:37 watch them work, right? And so I'll spend a couple hours and I'll go, let's go through this whole process and I'll write it down. I think we're gonna get a stopwatch and go, okay, you have a
38:46 bill of material come in, go. And then have them do work on the way they normally do it And then once, you know, ours surfaces to them, right? Go. And then we can start doing proper
38:57 calculations for this market to really consider the value that we're offering versus, you know, some made up value maybe that we think, right? It's awesome. The cool thing is that you know you're
39:06 delivering a massive amount of value from productivity standpoint, you know, we get things done quicker, which is gonna result in being able to actually answer more of these quotes, which is gonna
39:15 be more money, which means that they can actually grow because like you mentioned, they're not checking the prices. There is one more nuance, I don't know if we have the time. Yeah, go ahead.
39:23 So this is what's interesting to me. So obviously the sales and the use that use case is very interesting, so I absolutely love everything about it. But if you figure what's traveling from an end
39:34 user to a distributor, right? So it's a description of a thing, right? But that description of a thing has
39:43 the material inside of it, right? And so no one within this industry has been able to capture the amount of material being requested in the total market, right? So you're saying like, maybe like
39:57 it's 50 steel, 50 something else, right? And so if I'm a manufacturer and I'm getting this large bit of material, 10, 000 lines, right, I need to track not only what type of material is being
40:11 requested three years from now, right? So I can kind of stock up, but I also need to purchase nickel in order to manufacture my valves.
40:20 And so now with a large amount of data, you can start getting an understanding of, hey, you know, you've had 3 billion worth of quotes, you haven't been able to properly gauge how much nickel
40:32 you're gonna need in two years. Well, now you need to go on the nickel market and hedge against what, right? You start seeing how this works? So it's like they don't have that now. It's like
40:40 part inventory management, right? Right. 'Cause the worst thing in the world is to just have inventory on your shelves, right? So you can better use that for better, more effective cash flow But
40:48 then like you said, yeah, tying to hedging in the commodity markets. Exactly. Like nickel. That to me is interesting. That is really interesting. So. I hope you cry.
40:58 That would be so wild. Marty, this has been awesome. Like always dude. If you guys are listening to one of
41:06 the websites, industrialdatalabscom, you're on LinkedIn, so we can find you there. What's your email? Marty at industrialdatalabs. Cool, so if you guys have any questions, reach out to Marty,
41:13 he's the man. Take a second, leave us a rating review, share this with your friends or anybody think that they would enjoy this episode and we'll catch you guys!
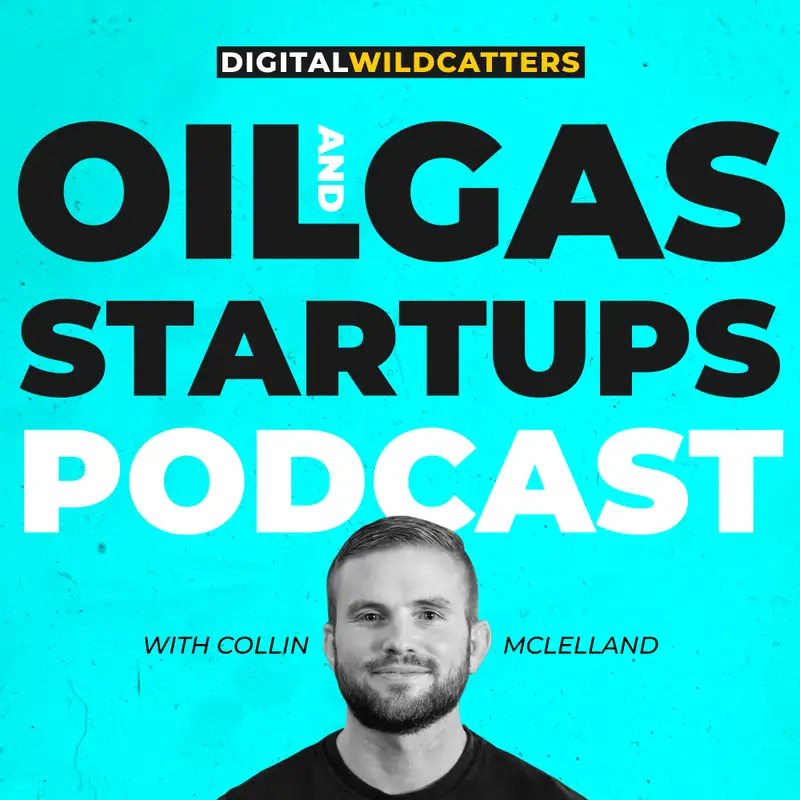