Revsolz Corp on Oil and Gas Startups
0:00 This podcast is brought to you by EnergyX. Are you tired of paying huge rates through the big cloud providers? Are you worried about being booted off a cloud platform? If your company doesn't meet
0:09 their ever shifting standards, ready to step up your data security and disaster recovery game? Well, ladies and gentlemen, your new cloud is ready. Introducing XCloud, the scalable, resilient
0:19 computing cloud that is also actually affordable. It's high performance compute for half the cost, HPC for HTC XCloud from Red Team is opening a beta program for new cloud computing customers, and
0:32 that means you, my friend. XCloud is powered by the XMDC, immersion-cooled modular data center from EnergyX. I've seen the data center operation and it is a total game changer. So if you want
0:42 more information about the beta launch, go to the URL in the description, type in promo code betaBETA for 50 off of your first instance. And so the URL is going to be digitalwalkcutterscomenergyx.
0:57 What is going on, guys? Welcome back to another episode. You know what? I appreciate all of you that have been listening for so many years. We were just talking about before we got on the mic.
1:05 And that's how long we've been doing this. And this podcast has been going on well over five years. And so a lot of you all have been riding with us since the very beginning. So I just want to give
1:12 you a shout out in this holiday season. A lot of gratitude. I appreciate you guys. We've got the guys from RevSolz in the studio today. What's going on, guys? Good. Nothing much. Glad to be
1:24 here. I'm ready to dive. I'm ready to just dive right in We've cracked open a little whiskey. Yep. It's a Friday. It's four o'clock, might as well. So high level, what is it that you guys do?
1:36 So, kind of our why is in the completion space. Okay. It's that you've got a four million L industrial process. We spend almost half of our well budgets on this thing. And what do we do? We
1:52 basically throw a bunch of sand and water in to a black box. and outcomes in production. We've really focused a lot on the timing aspect of how quickly we can put sand and water into our box, but
2:06 there's so much that could be done to make that process better. And we're really passionate about the fact that as we get to tighter spacings, we're losing the tighter acreage, we have to unpeel
2:17 that box and be able to look inside to make that process more efficient and maybe more effective, improving our MPV per, let's say square foot of stimulation. So really what we're doing is we're
2:33 basically deploying modern technology, modern computer science, digitizing everything, understanding what's happening to understand the process better, to enable the better results on the output.
2:44 So we're finding based on the principle that we're going to look at that stimulation problem. We're gonna try and really get everything digitized, apply technology to get better outcomes. So if you
2:54 had to summarize What is the one thing that people are missing? 'Cause you described it as much sand and water in, is into the black box and you get out the production. What are we overlooking that
3:07 is like the key that you guys have tapped into for driving efficiency? I mean, the big thing is the data quality, standardization, digitizing the data coming in. Like from the drilling space, we
3:19 did it from like PASON. It was quite easy because there you have the drilling rig and these tiny and it's good. Completion is the Wild West There's no even wit some melt for completions. There's no
3:27 standardization in completions. So the first thing is realizing that when you tap into a completion fleet, you're going to have to deal with unstructured data streams, structure them, deal with
3:38 like offset wells. How do I get that data? It's sitting in a SCADA system. We're bringing all the data together first, cleaning it, so we can use it and then applying analytics and other tools on
3:49 top. This are understanding how we can actually do better in that process
3:55 Yeah. Is this hardware? Is this
3:58 software? Or is this both? Everything. And is there a consulting component to this? Or is this your you sell the hardware software and you give the company's access to it? Yeah, I mean, Mark,
4:07 you sure? I can take it. So it's no consultancy. It's more of a holistic, I would say, approach where we are providing the hardware and the software and all the toolkits and all the necessary
4:24 functions, features, all the way from the diagnostics and analytics. So the customer client itself will be able to provide and generate their own conclusion of it outcome to get a better insights
4:39 about the job, about the path.
4:43 So it's
4:45 obviously the, behind the software, there's a data scientist, engineers, petroleum engineers mainly. And the software engineers, putting our as much as possible knowledge, experience, and
4:59 understanding so we can provide something automated
5:05 and clean the data sets for the clients to be able to manage their data and get their conclusions into predictions right away by themselves. So, like, it's like, pace on for completions.
5:15 Basically, yeah, if you want to summarize that the way, because really, when you start doing this, you need edge devices, so we do IoT boxes in the field We do connections to SCADA systems to
5:25 bring data in cloud computing. This is high frequency, large data sets that are coming in at very high frequency. No other type of system is going to work except for cloud computing. Yeah. When
5:37 you're going to deploy sensors in the field, that'd be wireless, and if they're wireless in connectivity. And then these data sets are massive and large, and when you haven't cleaned and
5:47 structured to drive insights, machine learning, artificial intelligence, when you start looking at this problem, you realize that you have to deploy the full toolkit we have of modern data science
5:57 in order to get to insights that you can use as a job's happening. What are your videos, what are your best backgrounds? How do we get here? I had a long slumber's head road. So I did a life over
6:10 there, saw 18 years in slumber's head before we kind of got into rough solves, did five years of wire line in North Africa, in Algeria, then I shifted over to Canada, I want to do something
6:22 technical, so they cut my pay and took my field bonus, and I started as a production engineer, then a reservoir engineer, then a simulation engineer. I crossed into drilling for two years, so I
6:33 worked in Tulsa for the drilling part of summer day for a while, two years in Brazil, where I was running a subsurface, a huge subsurface project there, and then it came to states in 2013, where
6:45 I was at the beginning of the shale downspacing revolution, and I worked in basically all kinds of business development Around that, integration roles are the product lines. And my last job, I was
6:56 running the full consulting C suite for Summer J in North America. I left 50 technical experts working under me doing all kinds of integrated projects around unconventionals. It's, I
7:11 was born and raised in
7:14 Baku as a bajan. I'm not sure if it is a well known place.
7:18 Probably the first person I ever met from Asia. There you go, there you go. Nice place though Very, very cool in a modern and old vibe when it comes to the city and the country itself. So I got
7:31 my bachelors over there and then I got my masters in Lafayette, Louisiana. I, in the patrol engineer, both of them. And then I started my career with an operator side. And it was with a major
7:44 company, BP. And I
7:46 worked in the multiple regions of BP, Caspin region, North Sea, Gulf of Mexico.
7:52 then I moved to
7:56 stone energy and it was in the Louisiana as well. They had different assets in the Appalachian Basin, West Texas, shelf assets in the Gulf. So I did lots of projects in reservoir and production
8:10 engineering and involved in the completion side of it. For the past six years, I moved into a Canadian side of it where I got my permanent residency and then the citizenship. So I'm the Canadian
8:24 right now and
8:28 I switched my career from the operating side to the service side of it. And right now in the service side, where I started getting involved as much as possible with the IoT technology and where I
8:40 started seeing lots of values, lots of
8:45 potentials when it comes to adding benefits advantages for the organizations.
8:52 started leading me into an
8:56 idea where we can put together the gap that is existing right now in the market, where we can standardize and digitize and provide the clean data sets for the clients. And we founded Refsols, and
9:11 Sam was one of our, I would say, initial members that joined the team at a very early stage of the company And since then, we are
9:22 pretty much penetrating North America, South America. And it's getting as much as possible visibility in terms of what is the issue and how it needs to be addressed. And that's what we provide.
9:39 Yeah, because from my side, all those studies that we were doing, it was always looking backwards, looking at the train wreck Yeah, and then you say, well, you should have done this or should
9:49 have done that. The completion should have been like this like that. You go one mile over and it doesn't work anymore. Shale's not like a conventional field where you have one full model, the
9:60 wells are all connected, the rock changes too much. So if you really want to get into the optimization space, you have to do it as the job is happening. And I was always looking for something like
10:11 this. And when I saw what Mark and the team were up to, it was kind of, this is exactly the tool. I've been kind of working on the background for a couple of years and they were just a lot years
10:21 ahead where they were with the IoT, with the kind of data scientists, software engineers that already had in place. I mean, we're engineers, but our team is 80 computer scientists or software
10:32 engineers. So, yeah. So when did you guys go full-time on this? 2020, July. That was right around the time we did. Yeah. Bad doing the course. Great time. We had left all the great things
10:44 that were a little bit more secure and made us money and um. Exited sold or shut down all of those things to go pursue this dream. Yeah, and then immediately it will go snake in thirty seven
10:59 Pandemic does right? Yeah
11:02 This is great timing. I was like God, I feel like such an idiot, but it all worked out It's funny how funny how it plays out. So did you guys bootstrap in the beginning? Do you guys go out and
11:12 raise raise any capital? It was
11:16 I would say you know seed investment. Yeah, I'll put it out angel investment. Yeah, right at the beginning and then I would say late
11:27 2020 Everything started with strapping and yeah, we received some grants here there from the government But overall everything was a bootstrapping. Yeah, and now we've got kind of a you know,
11:37 we're have clients lots of clients in place So we're able to grow out of our cash stream. That's perfect. Yeah, that's where you want to be It's it's hard. I'm not gonna I'm not gonna
11:49 I'll admit it's challenging in terms of having it funds available for you, you can invest on the technology, you can invest on the resources faster and get the product in commercialization sooner
12:04 into the market. But there's a risk too though, because again, you can take a lot of money from somebody, not have a focus for it and you can blow through a lot of money and not be further ahead
12:13 and you could be focused. So it's definitely a balance. There's a pros and cons Absolutely into that. Yeah, 100. So you guys go full-time. Do you focus on building it? Do you build the edge
12:26 devices or do you procure the edge devices? I'm guessing
12:30 you built the software. What did you guys build? What did you guys buy? So we built in-house everything when it comes to data science and the software. So the software package that integrates the
12:42 data science into it, it's our in-house built product. When it comes to the hardware, we're purchasing from the manufacturers, we assemble it in-house according to our needs and deploying to the
12:54 assets ourselves as well. So it's management of the operations is also on us and assembling is also on us. But the equipment where, yes, we are purchasing. And then the output is just dashboards
13:09 that people are using throughout the various stages in the completions process. Yeah. So, I mean, there's kind of three modules that we showed to our customers We have a fourth or machine learning
13:19 piece that we've kind of working ourselves. But really, the first one is the treatment, well-modern tree piece, which is everything about time. How well are we using our time during the frack,
13:30 right? You can set an objective that, you know, two hours per stage, but you can break it down further. Understand we've been the wire line data as well. So we can break down exactly which stage
13:39 is doing, how it compares to the next stage over, where the wire line runs consistent I mean, you can look at a job, for example, that was. Very successful. They finished 10 before it's
13:50 supposed to be finished. But you drill into the data, and you see things like the wire line pullout speeds on four stages were not nine minutes. They were 25 minutes. And you add that up, that's
14:02 two hours. You look, for example, on the pressure testing side. You should build a pressure test in a couple of minutes. You find four stages that pressure tested over 30 minutes, and there's
14:13 another four hours. So just by looking at the data and looking for key insights, you can realize that, yeah, we're 10 ahead, but still we still wasted a significant amount of time on the field
14:25 paying for it when we weren't being productive. This is typically the company, man. So we see all that data coming in, and then we basically have an interface that is easy to use and can use.
14:37 It's on a mobile device. It can be on a computer. So we give access to everybody. We don't charge by the user. We just charge when we acquire data We want everybody have full. visibility, full
14:48 democratization of that data. And then that's from everybody that you're pulling data from as well as towards being sent to. Everything, yes. Everything around that job, the company man can see
14:58 it and can interface with the software, easy to use, the engineer can see it as well. Everyone can see the same data sets all the time. Give me some example of the kinds of data that you're
15:07 pulling in. So, yeah, sure. So yeah, just adding into that previous one, it's all the way from the site to the office. With the cloud, we are giving the providing the access so they can mine
15:24 or their wells, they can see those key performance indicators and efficiencies on their dashboards all the time 247. And the data that we're pulling in is the FRAC data, which is coming from the
15:38 FRAC van, and we're setting our IoT device, industrial IoT device into the van, pulling all this information data. all the way from the slurry, propane concentrations, and send it to our clouds.
15:52 The same way from the offsetting side of it, where the surrounding wells started as - Your pair of wells, basically. Kind of both, yeah. And we're getting the pressure data, mainly out of it,
16:02 surface gauges, we're putting our surface gauges on that, and streaming everything to the cloud as well. And once we get into the cloud, the, I would say, magic starts right there. And actually
16:13 another exciting thing about the parent side as well, is that yes, run gauges, wireless, no problem. But because of the way we structured our cloud, we were tying into people's SCADA systems and
16:23 bringing their SCADA data into our system as well, which means that infrastructure they've already paid for, can be used to look at how the wells are interfering with each other as well. So we're
16:35 basically, instead of, you know, I mean, gauges get expensive, right? So it might only run like maybe 10 gauges. We can throw a huge net over the field, and monitor everything using the
16:44 infrastructure our clients, which is really saves the cost. So you're monitoring multiple pads at once. We can. Okay. What's like the most you've done? Wells, 40 Wells. Well, I would say with
16:56 the skater and the Wells, I would say 80. 80 Wells at the same time. Yeah. Yeah. From one pad. Wow. One pad and then the surrounding Wells. So super pad. Yeah. Well, it's the pad with the
17:09 six Wells or eight Wells, depending on how high it is. And then the surrounding Wells, depending on how dense it is. When like the one inside surrounded by all the other Wells Yeah, and it could
17:18 be 80. So you say once you get all the data together, that's when the magic happens. Let's unpack the magic. What is the magic? You wanna go first? Hi. Sure. Go for
17:32 it. We talked about the KPIs. We talked about the efficiencies and that everything comes from the frack side of it. And all those automation that we have applied from the data science perspective,
17:45 the flags that we are highlighting, the breakdowns of the activities within the operations, everything goes into the charts, plots, tables, so you can see and see the correlations and see the
17:59 discrepancies between one stage to another stage. Yeah, 3D as well, 3D dramatically. Yeah, and adding a 3D visualization into it is becoming more granular information about your pad itself, how
18:13 this one stage is compared to another one. That's from the frack side of it. When the opera, from the offsetting side of it or the parent, well, it's becoming more of alarming and putting the
18:26 smart alarms where you can put, you know, thresholds, slopes, and differentials, and get alerts and get the push notifications, whether it's your phone, to your email, or even buzzing on the
18:39 screen. That gives
18:42 instant alerts where where you can prevent and do some defense mechanism or reactive approach to your wells. And then the last piece where we are trying to marry these two data sets together and get
18:58 some more diagnostics and analytics on top of it. And we're talking about the interference analysis, interference between the frack well and the offsetting well. The pad that we're talking about
19:10 with the AD wells has to, I would say, maybe most of the time, you know, 50 of that could be in communication with each other. And that communication is, most of the time, it's not desirable.
19:24 And when we see this in interferences, it could be causing your production impairment or well-word damage, integrity is the issue. And in order to avoid all of that, there's no tool out there that
19:38 is going to do everything automatic that for you, pick an oldest event. all these interactions, the degree, the magnitudes, intensities, slopes, and then giving you some insights about how you
19:49 are performing from one stage to another, and what is your communication degree? And if that communication is not desirable, why we're continuing putting that much energy down to the well? And it
20:01 becomes already a cost issue because the
20:06 MPV return on investment of what you have pumped. And you can see it live, instant. So you guys are going, are you guys going into the financial side and actually giving?
20:16 We're not, I mean, we're not there, not there yet, because the financial side means getting into someones financials, which in oil and gas is fairly protected. People don't want to tell you what
20:26 the true cost basis and what their return is on their production. But we can provide them tools to basically understand when they're having or interactions which they can use to then basically make
20:37 better decisions about what to do when they're cut stage, keep pumping, or even just to learn for the next pad. So you guys, are you guys focused specifically on the completions stage? Are you
20:54 guys hanging around once it's actually in production with, with skita?
20:60 I would say primarily during the completion. Okay. So from the start of the completion till the end of the completion, we are on that, on the, on the pad and we're watching offset balls and the
21:10 frack balls And then the, the cracking down and getting this insights about the interference, if there is any interference, once everything is done, obviously, everything needs to tie it in at
21:25 some point in production. How my production is doing based on this interferences and, and that we have, we have that ability to plug into production data and analyze the production data, post them
21:37 and, and, and, and pre,
21:40 pre this. the job and then analyze how much rates we have lost and what is the reserves. So all of that is possible and get into the commercial dialysis as well. I mean the discussion we had kind
21:55 of before we came on about, you know, before the guy to manually edit all the cameras and now he does it automatically. In this space it's the same thing. This interference analysis would take an
22:06 engineer weeks to do this. Two to three, it was typically to pick all these events, mark them all, you know, I kind of line them up and do it and now we provide that instead of someone happens to
22:16 do it manually, it's being done as it's happening instantly. And the consequence of it being a two to three week task is that most companies know it was valuable, but unless it was a specific pad
22:29 with a specific problem, it wouldn't be done. But this way you get a database of statistics about what's happened that can really used to drive learning going forward. I mean, this sounds like
22:39 it's Relatively, it's an easy concept, but very complex to actually pull off in reality. What have been some of y'all's biggest challenges that y'all have kind of faced along the way? I'll take
22:51 the first one in the long past. The biggest one is actually this is truly a big data problem. It's a computer science problem, Silicon Valley problem You're trying to use all these tools, edge
23:06 devices, cloud computing, AIML. We have, like say, 80 of our staff are doing that, but we didn't try and hire petroleum educated people. We found the best people in those fields and the
23:24 educational process is a challenge of finding people who can bridge that gap And, like I say, the reason we're doing it so fast is because we really feel we have such a strong background about
23:36 software and data science. with our knowledge about oil and gas has allowed us to develop quickly. But that was a huge challenge, I think, the people aspect. And I would say,
23:48 you mentioned that at the beginning, right at the beginning. The data quality, the data standard. Or lack thereof. It's the
23:60 huge issue in the completions. Just because, you know, we're investing a lot. We're investing a lot in completions Drilling part of it has been explored and being into optimized optimized levels
24:14 at this point very well. When it comes to completions, we're spending lots of millions into that, billions into it, but at the end of the day, we are still, don't know what's happening, and
24:24 everything starts from the data. If you don't have data in your system, in digitized, standardized, synchronized, and clean data set Mm-hmm You cannot do a lot, and that is one of the challenges,
24:40 and it goes from one frat company to another frat company, everyone sends different type of data formats. So you need to standardize all of it. You need to clean all of it. On our side, I have to
24:51 standardize it. And we are the ones, not only us, but there's a few companies are tackling into the same problem. We are trying to get into
25:02 details of it, how we can make it apply to all the frack companies. Like drilling has Whitsemel, for example, in a standard, every drilling company uses the same language. Like the only thing
25:14 standardized from a data perspective in the entire industry. Yeah, yeah, yeah. It's, once you have, that part solved, you already like 50 solved the problem. And then the rest part of it is
25:27 just a crunch in the numbers and seeing what the data is telling you. So that part of it is already a powerful machines that are out there and you can apply everything on the cloud and get your
25:36 results. Yeah.
25:40 What do you think, is this something that you come in whenever you're presenting to prospective clients? Is this something that
25:48 sells itself? Or is this something that there's like, there's convincing, like, dude, does everybody agree that there's a problem with this? Or is there something that sometimes you kind of have
25:56 to overcome a little bit of a hump in terms of showcasing the value? Interesting, yeah. I would say that everyone agrees there's a massive problem. But what does the data mean? What do these
26:11 interactions mean? How can we, you know, get confident enough to make changes or even change our planning? That's the real hurdle because it's been a black box for so long that now that there's
26:25 data out there and we can do it on a scaled, you know, field wide type of level. Now it's basically the, the, um, it's not education that data, the data is good. It's more just how would I
26:39 change my process because in, you know, like the unconventional space is very process oriented So it's kind of how do you put the interaction scene wells into the planning process and also in the
26:52 operational process. But the actual value of the data itself, everyone knows it's valuable. Everyone's tried to do it. It's just we have done it. We've always done it. We put engineers into the
27:02 process. We kind of hard coded. We kind of do it manually. And now the tools are available to actually scale this. And we're basically in the scaling business, not the, you know, just the one
27:13 off interpretation business Yeah, so for so long, nobody had asked us all this data, so now everybody's like, whoa. Now we have access to it, but we've never really had an experience of like
27:21 actually taking and interpreting and figuring out what does it mean? Because you guys said it's just like massive, massive amounts of data. But I mean, there's like an example, two from this week.
27:32 Um, number one, Permian Basin company wanted to base it. Thought they had a barrier, thought they could put well above it and basically get another bench and from our data sets, it was pretty
27:43 clear that that didn't work out And the investment in that was in that one pad was, you know, a20 million test of the idea. But they were planning to do more of that. So this was a way of quickly,
27:56 you know, of proving with other data sets that that barrier didn't hold. Another one from the Eagleford, you can see from our data sets is a clear fault running through the pad. They didn't
28:08 realize there was a fault there and they laid their wells into the wrong landing points because they didn't notice And this pad had happened four years ago, well, three years ago. And even now
28:20 talking like, oh, now they're going back and looking to see if there was where the fault was across that section. Three years after the fact, every other well laid in across that part of that
28:30 reservoir would be hitting the wrong target point because everything shifted by 25 feet. So those are material100 million type of things that just come from illumination. We're very good with data.
28:45 But as engineers, we need data to work on Yeah, obviously there's been a rise of AI over the past, particularly last year, especially last six months. Is this something that you guys see in your
28:59 roadmap in terms of implementing? Like, does it actually make sense? So like, because you're capturing data from the source, going to the edge device, you have this unique opportunity because in
29:09 theory, you have this clean data. Whereas if I was at the same conversation with somebody that was managing, say, production data or just company-wide data, there's a lot of cleansing that would
29:21 need to happen to even take place, right? So is there any applications that you guys are thinking of there or is that like kind of a pipe chain with this plan?
29:33 Short answer, yes, it has been on the roadmap from the beginning, I would say from the start of the company, we are truly believers when it comes to the statistics and when it comes to machine
29:44 learning AI, that's the only way, that's the way you can get to the answer. Every company, I would say operators are doing lots of physical modeling, numerical modeling. They also apply some
29:59 statistical approaches and I do see and I would say overall the
30:07 company, the core team is seeing that machine learning is gonna get us to the right solution, right answer. And how we're going to apply it is really going to be a intuitive process. But at the
30:21 end of the day, you can find the correlations. And we do see the correlations already from the frack to the offsetting wells, from one path to another path, from one well to another well. There
30:33 is some indicators that we can see the pattern that is happening. And all we need to do is just get it together, put it together, which we are doing already And get into predictedness of it. So I
30:48 mean, really kind of what we're looking at is, you can imagine we're getting all this data about wells at different spacings, different treatments, different depletions around the wells. We can
30:59 invert the problem, which means that if you say, I need to lay my wells here, which you can't really change because the lease line constraints, you're stuck with your spacing, basically. You
31:09 know the depletion, then you can actually like a planning flag. on these wells have an issue with communication, and then you can play with the toggle, which you can control, which is your
31:19 completion. So we're actually actively looking at that, trying to invert the data to predict before it happens, because we have so many data sets now about when it happened.
31:32 What are you guys most excited about moving forward? Me? You. You go first, I'll go ahead and say, I know exactly what it is
31:43 There's several, I would say, sides of the excitement. It starts all the way from first engagement with the clients. When you engage with the clients, 'cause there's so many ifs and dens when it
31:59 comes to what the data is telling me. And you don't know how the conversation is gonna go. You don't know how it's gonna continue. And that's part of the excitement that happens. on a daily on a
32:13 daily or a weekly basis. But when it comes to future, I really do see how the completion world can change dramatically, drastically, and, and, and we're close to it. And if that, if we are
32:29 gonna get, when I say we, I'm talking about as an industry, if we get to the point, once we crack this puzzle, all, all is going to happen is just a
32:44 continuous chain effect, and we're gonna see how better wells, low costs, reduced costs that are gonna happen on the wells, on the, on the pads, higher performances, productivities is gonna
32:58 increase. So that excites me a lot, and we're not far away. I'm really believing that it's going to be in this decade.
33:09 For me, maybe even more general. For me, what excites me is that getting so close with clients now, they can see the inefficiency. They can see there's ways you can see these things happening and
33:21 identify that this was really close to my well and this was fully connected and it didn't go anywhere else but into this well that what really excites me is getting to the point where as an industry,
33:33 we realize that we can change. We can change our completions We can actually do things in the black box process to make it better both from time and both from cost and from production. What really
33:46 excites me is finally getting that level where getting at least one or two of our clients to really push to the next level which is realizing that a completion plan can change while you're pumping and
33:59 that's okay to get a better result This is super exciting. There's not many people that have been focused on this space period. And so it's really cool to see what you guys are working on. so glad
34:12 to have you here. If somebody wants to reach out, if they're like we have an absolute disaster in our completions phase, we need you guys to come and help us. Where do they reach out? There's
34:25 several ways.
34:27 The first place is going to be our website. All the contact information is listed in our website, which is going to be refsalescom. And then it's going to be one of us or our sales team, which is
34:42 led by Sam Rogers. And I'm not sure if we need to provide their contacts here, by the way.
34:51 Yeah, you guys are on LinkedIn too, so they can reach out to you as well. Yeah, Sam and Roger are linked in 832-540-4254. We're available. We're like on every social media where we're just just
35:03 go to Google and put RevREVSOLZ. You'll find us.
35:07 We're we're yeah, we're everywhere. Super upsetting, guys. Thanks again for taking the time on a Friday late on the Friday. Hey, we got some whiskey out. Yeah, it's good. Um, this is
35:16 fascinating. And so, uh, excited to keep up with you guys and see how this continues to evolve in your seat. Yeah. Yeah. Appreciate it. Thank you. If you guys enjoyed the episode, take two
35:23 seconds and share with all your colleagues. Send this to your entire completion department. Let them see it. We'll catch you guys on the next episode.
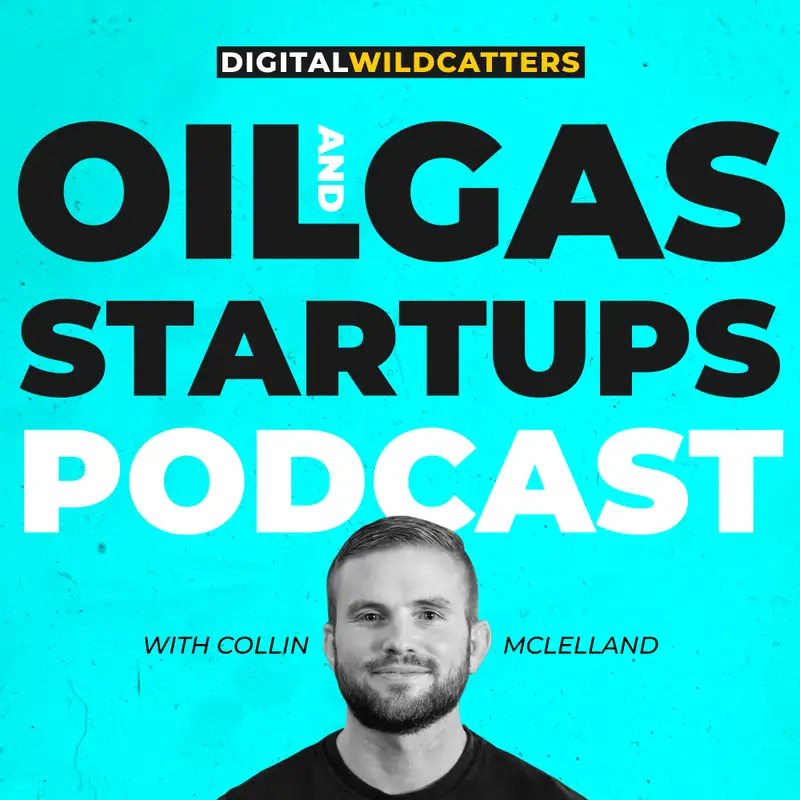