Amplified Industries on Oil and Gas Startups
0:00 What's up, guys? Welcome back to another episode of The Willing, I started with a podcast. We've got the guys with Amplified Industries here today. Y'all changed your name, right? You see
0:08 acoustic oils before? Yeah, that's right. That's right. I was, I was trying to remember you guys came by God. It must have been three years ago, I think. We were on the other side of the
0:17 building, told us a little bit what you're working on. I know it was kind of early stages back then, but I'm excited to kind of dive into what you guys are working on now, how things have evolved.
0:27 So really quickly for everybody listening What is, what is Amplified Industries?
0:33 Sebastian here, so you have the company. We build the next generation of automation and control system for the old field. Okay. So at the well side, at the well side, from the equipment on the
0:44 wells to the math running on the cloud and anything in between. And on top of that, not just like your device that's going to go physically there, the old data pipeline that allows you to get that
0:56 at a very high resolution, for high quality, all the way to a cloud. process into actionable insights. So is this more like on the production optimization? Exactly, yeah. We help the production
1:06 engineers mainly today. So you have, you know, your guy in Permian who's in charge of a couple hundred wells, put the system there. The wells are gonna run, you know, as much as possible. And
1:17 it'll pilot basically with the system and then he can dive in and use his expertise. Do you reel in depth, understand what's happening on every well, especially on all the new wells, is all kinds
1:27 of complicated flows It's basically that happened because of those big horizontals and lateral legs, and that's where we come in. We allow him to get this big picture of you, dive in where he needs
1:40 it, and let everything else run automatically, let the system do his job where he doesn't need to dig in. And at the same time, the guys in the truck were running their routes, making sure that
1:52 everything's going the way it should be. They have our app, and as soon well goes down, Stop pumping, they know about it. They lose pressure on the flowline, they know about it. They have the
2:02 tank that's full, they need to get sips. To get sold, they'll know about it. So both in the office, like high level, doing very, very knee degree technical deep dive, but also at the
2:12 operational level, making sure that everyone is aware of when something bad happens and can be on top of it and go fix it. So kind of like this pump by exception thing that we've been chasing for a
2:20 long time of, like just being able to know exactly what is going wrong, be able to almost like create tickets or what to suppose and literally dispatch the pumpers there and then work hand in hand
2:31 with the engineer. It's kind of like behind the scenes to optimize production. Yeah. That's one of the interesting thing. You have all those layers from, you know, the foreman on the ground,
2:40 the engineer in the office and more the executive guys that want higher level, you know, different analytics, different visualization for a different job position at the end of the day
2:51 and building that out there for
2:56 different kinds of company from you. small mom and pops to your larger independent producer. You know, your company with a nice tower in Houston and anything in between. So what are those
3:08 backgrounds again? So let's see, depends how far you want to go there. You want to go back first? Yeah, so my, I'm French, I've been here since 2012 in the US. Okay. I used to be in quantum
3:22 physics in France and then in aerospace, ended up pretty randomly honestly doing an internship with Schombre J here. They had their research center right across from MIT. I was like, why not? And
3:33 I loved it. I was very impressed with the work I did over there, ended up doing the whole PhD with them on pump jacks, model the flow, the waves, the sound of all the physics that happens on the
3:43 ground, gave me a few ideas of my own and decided to start a company. So I kind of blended a lot of the, I worked with Siemens on turbo machinery, I worked aerospace company before and build
3:56 bringing all those mindsets to you know, you would field in the upstream sector. So what, okay, so that's wild to me. What was like the allure of, was it SLB as a company or did you become
4:07 completely enamored with like the industry? 'Cause I mean, if you're coming from like aerospace, that just sounds like the coolest industry in the world to work in, in my opinion. So like, what
4:16 was like the draw for you? The draw was, I like big industrial toys, right? Like yeah, kind of classic mechanical engine, you're mind-set, you know, you make an impact and all those, those
4:29 large machines. And Schlomerge was, is an extremely high tech company. I like, we were building miniature particle accelerator to put in tools that are brought, you know, down hole. And I
4:41 didn't expect that. And through them, I got to go discover all kinds of oil field. And I just loved, and the first time was in the, some Oklahoma fields in the South, and I just loved going
4:53 there. Like talking to the pumpers and all that And it was the most, you know. extreme difference between my experience, which was like in France, I was in the rocket engine factories for Arianne,
5:06 like sterile environment with like two guys basically surging, assembling something in detail to like some scorching field in Oklahoma with the pumper and his cigarette.
5:20 Very different, but at the end of the day, the oiling gas industry deals with real important problems. And
5:29 that's why we wanted to help. We saw a need for it technically speaking. We saw that there was also a change of generation coming, frankly, there's a bunch of new engineers and generation like you
5:42 guys that are a lot more open to change industry and adopt new technology and all those buzzwords AI and so on. And he decided to be part of this. Do you come from the industry as well? Yeah, so
5:55 my background is somewhat similar. So I'm also French. Seven, I actually know each other from at this point, 15 years ago on the grad. My background is initially very mathly and physics. And I
6:09 sort of had this oddball class when I was in college about basically energy. And very focused on making us realize how central it is to basically modern civilization and like energy is a lifeblood of
6:24 everything. Like there's the throne product everywhere in this room. Like this is plastic, it's the throne. Yes, synthetic fibers, petroleum and all that sort of stuff. And initially I wanted
6:34 to go actually in nuclear engineering, that was the kind of stuff. I was more coming from this fixed background. That was exciting. Pretty quickly became clear to someone. Well, a little bit of
6:45 foresight that the French nuclear industry was not going anywhere And so I figured, OK, so slumber just had this really good reputation as like - excellent top tier technical company.
6:58 That was, and I figured it out. I'll go to an internship there. I'll be in the sort of like the engine of the world. And it was 2012 first time I worked in the industry. And I mean, it was a
7:09 crazy time. Like it was Pico everything. The Shell revolution was not, was starting maybe to happen in the US, but everyone was looking for the crazy next place from which the next barrel would
7:19 come from. So, Arctic project, deep offshore, pre-sulting Brazil and eventually Shells was restuck. But so it was there and I've realized it was kind of the same thing basically, really, really,
7:32 really
7:35 technology intensive in some ways. But then I got my PhD in math working with Total which is a French oil major. It was interesting because it was oil and gases is a weird place where a lot of what
7:46 we'd consider computer science digital technology come from there. Like high-performance computing, It's, I mean, the people who made it are basically either nuclear bombs simulations or reservoir
7:58 simulations, this kind of seismic seismic, like the drilling technology, it's like crazy, like rocket science literally. And on the other end of the spectrum, like a lot of the more
8:12 like user facing digital stuff, the automation system, all of that is super old school. And so really got interested into, okay, how can you, this is a big industry, the very big impact that's,
8:27 I mean, gonna be with the world for a long time. And as a lot of responsibility and stewardship to
8:36 own basically. And how can you use like those new tools that have starting to come from other places and bring them in this industry, which is dealing with this massive problems, super interesting
8:49 systems, to be honest, like if you go to a drill location, like. any production facility it's like. Okay, that's real interesting stuff going on. And how would you bring all of those
8:60 technologies to this industry? So I've worked reservoir with slumberger, my PhD was more in process optimization on the downstream side, did some work on offshore drilling when I was at MIT and now
9:12 production optimization. Nice. So how did this, how did the kind of concept come up? Was it just seeing a need for this at SLB and just being like, well, if no one else is gonna blow, we will.
9:25 So the concept didn't come from SLB. I got exposed to the industry while at SLB and going on some field, I basically saw how oil wells are controlled.
9:40 And frankly, yeah, for we could do much better, right? Even on the first most, you've opened a couple wells, I think, most small wells in the US is just an on-off timer or some rustic system
9:51 like that That's ours, bro. Yeah. You get the breaker boss, so you can put the power on and off, and you get a timer there, keep it on 24 hours a day, you can set a schedule for it if you want
10:03 it. That's a couple hundred thousand. Very rudimentary. Yeah. Yeah. Next year up, you have a standard controller by the legacy guys, and if you time travel 25, 30 years, you will see a
10:16 different pickup truck on the well, but the exact same instrumentation like exactly, and in the last 30 years, technology has evolved tremendously, and so we wanted to bring all those new things,
10:30 and we thought we could make those wells produce better, detect all the leaks, control the wells, deal with the gas issues, and so on. And so we decided to build the system, and we saw in
10:42 parallel the industrial automation in general not just for Odfield, isn't what it used to be like to Charles Point. Up until the, it's called the 80s or so industrial system or top notch in the
10:56 most high tech thing you could find pretty much anywhere. Nowadays, the phone in my pocket is significantly better in pretty much every way compared to industrial system. It's easy to use. I don't
11:07 have a 900 page manual to deal with it. It does way more computing. Does it seamlessly as a very reliable piece of hardware? And you can put apps and put, you know, do anything with it, right?
11:20 It's a platform you can deploy stuff It's crazy. I just got the new iPhone 15 Pro a couple of years ago. There are apps on the camera side that compete with these very expensive cameras. Yeah,
11:31 exactly. It's astonishing. Imagine that on the node. Well, like the difference between, you know, a well controller system and a skater system in that you're beaming. You know, people talk
11:41 about all those words of big data and AI and whatnot. This is beaming back to, you know, Apple and Samsung and whatever app you're huge amount of data about your behavior, about what you're doing
11:54 with the phone.
11:56 You're talking about a million times more than what the industrial system beams back to the headquarter of a company. And because you have so much, then programmers, computer scientists,
12:05 mathematicians are able to build those cool apps and cool application. And so how do you do that on the field, but taking into account the reality of another field, which is stuff breaks, you're
12:19 in tough environment. You can't build a crazy system that's going to end up costing an arm and a leg, because most of the operators we deal with, they have between 100 and a couple thousand whales.
12:32 They're not going to buy a 50 grand system times 1000. We focus on
12:38 PDP. Those are whales that have been drilled already. People already spend their multi-million dollar to drill and complete a whale, and now you need to produce it. So you work on a much tighter
12:48 budget, but your work at scale. So how do you make? those technology accessible at scale. And that kind of led us to develop our own hardware. It's something that is not very trendy in the
13:02 startup space. People try to, as much as possible, stick to your software. Do you all think you were gonna do hardware from the beginning? Or do you think that's gonna be pretty much? No, we do
13:10 from the beginning. The, you know, the little bit of a cliche example I give, but I have in my apartment, I have an AC system, right? And so, big manufacturer, and I have this beautiful
13:20 remote with 90 buttons on it, right? And I use onoff and plus and minus. And this is the reality of industrial system on the wells, right? And then your foreman or your pumper is stuck with that
13:33 machine that has a gigantic manual. And at the end of the day, you just want the equipment to run. So we knew we had to design something very simple that was easy to deploy, but that would, at
13:46 the same time, be high-tech enough for us to be able fancy analytics that we wanted to do in the back end. And we saw a need for it because the business model is also very different. Big automation
13:58 company, you make money by selling you every 10 to 15 years, a new expensive box, right? You have the box 10 and 12 year later, you bought four. You buy for another 20K the, box 20 and so on.
14:11 This is an our approach. We make money by providing a service and we want everything to just work. Like if I can instrument everything, you know, for you, I will. The value comes from the
14:21 control, from the analytics, from, you know, people don't care about the hardware. They just want the well to run. The hardware is effectively a necessary item. That's a means to an end for
14:35 the analytics, right? Exactly. So it's a little, you know, controversial for me to say because I'm the one in charge of hardware in the company. You have design all that. I think it's extremely
14:41 important you have it, but if I do my job correctly, the hardware will be completely transparent.
14:49 something like, I mean, we were talking a little bit off record initially about why AI is obviously top of mind for everyone.
14:58 But I mean, having worked with big companies and big the guy who comes in and okay, we're gonna do some machine learning or we're gonna do some industrial AI project. Okay, well, so you go talk
15:11 to the engineers, we know about the process, you try to find prospect where where where is there an actual use case that we that can make sense for you guys. And like very quickly you run to
15:22 situation where you start with 30 guys. And every time you think you found something that's impactful, that's that works. And okay, do you have the data? Actually, we don't. Or what's the
15:32 resolution of the data? Oh, it was compressed to one point a day, or Oh, actually, the the layout we have for for the way everything is wired, no one can is completely wrong. And So very often,
15:46 like, I think if you want to like something which is very important, which is happening is you need to have like those layers of people who come in and will basically help you to scrub all of that
15:56 stuff and to organize it. But if you want to build like really high frequency application, you need to like there is a bottleneck on how much data are you acquiring? How hard to do it? How messy
16:11 is it? And how much of it do you get back? And the reality is at the end of the day, if you want to do like there is a lot of value to be add by just you know, having clean clean KPI, not super
16:23 sexy stuff, but just thinks well organized to keep track of okay, at high level, you are my problems. But if you want to get into the more sophisticated workflows, like the kind of stuff that get
16:32 people excited about, I don't know, predictive maintenance or up production optimization, a lot of this stuff, you really need to unlock to unlock that whole pipeline from. What's the, what's
16:46 the sensor taking the data? How much am I losing when I'm transmitting that back and how much am I losing when I'm storing it and if you don't if you don't do that very you you you end up trying to
16:57 create like, you know.
17:01 You end up trying to to build a rocket, but your engine is from the 19th century. Yeah, so, yeah, not so far. So zooming out, you know, skate is not a new concept in a long time. There's been
17:12 some market leaders in the space for a long time Like you're saying, some of that is now more antiquated technology. It could be a lot more expensive. Want me through how you guys are doing this
17:23 differently? And I'm assuming there's probably costs, probably the fidelity of the data, the frequency of the data, things like that, leveraging some of the newest tech. But also talk to me
17:32 about what are the things that you're pulling off? I'm assuming it's probably speed, stroke, I don't know. temperature, I don't know if that plays into it, but like just walk me through how you
17:45 get to do it differently and then what you guys are actually capturing on the hardware side and then let's dive deeper onto the software side. So to be very specific, we capture on the well,
17:59 our kit is a few different sensors are placed around the well, little devices that talk wirelessly to one another, each recording, you know, a different sensor, like a hundred times a second.
18:08 Yeah. Compared to, you know, SCADA system is a point every 15 minutes. So when you record - That's okay, so it's a bad comparison then. Yeah, yeah, yeah. And, you know, inherently it's the
18:18 same way. It should be the same, but just there's a lot of constraints that come with what people traditionally call SCADA. When you go for a hundred times a second, you point every 15 minutes,
18:27 you could talk about, you know, 10, 000, hundred times a thousand more data. Your cloud infrastructure is completely different at that point. And by the way, the bulk of the work we do is a
18:36 cloud infrastructure, because it's easy to say, yeah, I'm gonna build a cloud, building an infrastructure that can at scale, handle in real time flow from thousands of devices at that resolution
18:46 and then process it each at a different speed and so on. That's where a lot of real know-how is. And that's the limitation with the current system. They're just not built on the, you know,
18:56 technology that support that, not even close, right? So,
18:60 but you go back to your question. So on the well we measure, for example, your tubing pressure, your casing pressure, the sounds in the pipe, we measure the force on the well, we measure the
19:09 vibration, the movement of the well, the current and the motor,
19:13 thing that's about it on the well. So, realistically, anything you need to know what's happening on the surface and what's happening down the whole. And so, from those measurements, you can tell
19:26 down the whole, you know, how much gas there is at every single stroke, your traveling valve is worn, your tubing is leaking, your, you know, whatever, your stuffing boxes is
19:37 due to it and so on.
19:40 And with that, you coupled that data with the other devices on the field. So we instrument the tank batteries, the injector wells, and so on. And that's something that sounds very obvious, but
19:53 is not a common practice at all, tying what's happening between the well and the tanks. Very often, it's basically the part of the schedule. The thing is streaming, yeah. Yeah, either the
20:04 pumper will gauge the tanks by hand and while the production engineers deal with their well data. And they're literally connected by pipe, right? Throw the chain down, or if you have a massive
20:17 stick, there's still different ways you can engage them manually and so we can go out and be like, oh shit, we have a little bit of a leak on a valve, but we wouldn't know. We didn't have any
20:28 automation on things. It was all completely manual, and the only way you even know that your tanks were full is 'cause the pumper told you, and what they were called in the run Yeah. That's all
20:38 about right. It's wait until transfer pump fails. Yeah, exactly. Yeah, you deal with all the saltwater injected. And basically by combining what's happening on all those pieces of machines
20:50 together, you can model everything that's happening on the well. You can tell this well is pretty in this much oil, that's much water, it's going to this thing and so on. And you can do that with
20:60 actually very few sensors. You can, you know, if you have what's happening in the well, what's happening in the tank, you can calculate everything after if you have the right amount of data
21:09 'Cause the traditional approach is a little bit you brute force everything. So okay, I'm gonna put a Coriolis meter, multi-phase flow meter everywhere. You know, there's a 10 grand of sensor here,
21:16 10 grand of sensor there, maintenance and so on. And before you know it, you have a system that is extremely expensive. So how do you do that with as a light, hard work footprint as possible,
21:26 but with as much computation after, as possible to deal with all those details.
21:33 And so it's doable, we've done it, right? you have the system I mentioned to you on the well. similar system on the tanks. You can detect all the leaks in the flowline in between. You can
21:44 allocate production. You can detect download. You can pretty much do anything that is needed. There's very few failures I can think of that we can't identify. We've seen early on, there was a
21:57 learning phase. Obviously, we built a sim like, oh, what is this? It's like, oh, yeah, it's because it was raining today. The belt was slipping. So the motor burns a little bit, the rubber,
22:05 until the water evaporates and then it grips the belt and the whole well will start, you know, like go figure when it's the first time you see that signal, that this is what was happening, like
22:15 there's no way. But if you have multiple signals at the same time, I'm like, okay, I see there's vibration on the wellhead and it's moving a little bit up and down. I see that the current on the
22:23 motor is doing this thing and now I can build a little system that will detect, okay, you need to go tighten the belt on that well, for example. So the bigger picture thing is like, it really
22:36 doesn't matter what, you're able to do today. What matters is what you will be able to develop. If you have all this data, you can just continuously release a new feature, release a new model,
22:46 either ourselves or the customers access to data and can build in-house stuff. You allow this feedback loop, this learning loop to happen. You're not stuck with the system that, oh, it can do X.
22:57 Oh, you also want to detect Y by the new box, 20, which you're never going to do in the real world. So you have a system that can evolve the way you update your phone. Do you pull in power
23:10 consumption? Yeah. Because that's really interesting, especially when you're talking about not only at the well site, but particularly on the solid or disposal side. Like, is that something that
23:20 you guys are actually able to optimize for? Because it would be a thing, but that's one of your biggest Eloise, right, is actually power, which is due typically to not only the well site, but
23:29 the solid or disposal, if you have it, right? So the main thing we do there is It's region dependent depending on which contract the operator have signed with the power company. But there's two
23:41 big things you can do. One is you can switch your contract. You have a time of day use. Instead of paying a flat rate, you pay a variable rate per hours. But then you can tune, depending on your
23:50 well, you can tune them to run more at the middle of the night, basically,
23:56 which by the way is the exact opposite of what a human typically does. 90 of
24:07 Foreman's set the wells to run when they do their route. So between 5 am. and noon or something, if they don't need to run the whole day, versus that's typically electricity is very expensive in
24:14 the morning and when people are home, right, early morning and end of afternoon, early evening. So you can do the opposite to the system. The second point is what's called a demand charge. Your
24:25 power company bills you based on how much kilowatt are you burn, how many joules of energy you're using and what's the peak power you do And, uh,
25:33 a little bit far off and you have to bring a truck to remove the water.
25:40 How do you know, how do you know exactly how much water was taken, was old by that truck and that's what you get charged for? How do you know that maybe the truck just took a half load but you paid
25:51 the same price anyway? All kind of little stuff
25:56 like that. There's a trust system based on tickets. All kind of like quote-unquote things, but if you want to, again, if you want to make things happen at scale in real life on the field, just
26:09 being able to see, okay, the truck came and he came at the right time and we had a full load of water to dispose of. All of this stuff, like we're a customer, we have saved a lot of money by just
26:20 basically telling the truck to the company, stop coming every Monday, just like, I'll call you when it's ready and you'll come pick it up What have the results been so far with some of your
26:32 customers? On the optimization side. Yeah, excellent. So we have very different kind of customers. We have some people that have, you know, the oldest well I think we're on is 1917, 1918.
26:44 Vertical wells. Still going strong. Yeah, yeah, yeah. Self down the earth. Old school too. I love our vertical wells. It's so pumped that you're dead. It just never stops. To our newest
26:55 wells, our early 2020-ish, horizontal big wells in the Permian. So, and then the thing in between, we have 14, 000 feet wells. We have, what's the shallots? We have, I don't know, 1,
27:05 000, 1, 500 foot, maybe. That's super shallow. Yeah, yeah, yeah, yeah. Water floods to, you know, horizontal to all that stuff. And different people look for different results. The main
27:19 thing that people want is production increase. And that'll happen on average, all around all our customer, around plus 7 ish of production increase across all the wells. 7, 7 Yeah, at 80
27:31 something barrel, that's a lot. The second thing is around, depending on the operator, either using failure rate or basically cutting LOE or finding spills. So for example, we have some people
27:44 that are in the swampy region. Louisiana, Mississippi, a spill there, you know, reserves. It's measured in millions of dollars to find you're gonna get after. So yeah, really don't want your
27:56 machinery to leak.
27:60 Other places when you have a well that's, you know, deeper than 10, 000 feet. Maybe you don't want every last drop. Maybe you just want to never have to fix that well because you know, the rig
28:09 crew is gonna cost a fortune or they're backed up and you're gonna have to wait three months for them to be on site. And you can operate so much leaner if you just, if you were able to do some sort
28:18 of like pretty domain instance that I haven't worry about blowing something out and lose all that.
28:25 LA and PT was not production, no revenue. We saw it that the last freeze, I mean, this winter was relatively pretty mild, there were a couple of cold waves and you see literally ice starts to
28:34 form in the pipe, how the pressure slowly change and so you can alert people, say, oh, this is, you know, you may wanna turn off all the machinery and play it safe 'cause nothing's heat-traced
28:44 over here. On
28:47 the other end of the spectrum, you have some people that have a horizontal wells, it's having a horrible gas log issue in very complex pumping condition, help us figure out, build an advanced
28:57 control model to run that well, what do you do? And then we have, you know, all the data, we can determine what's happening, we can try different control strategy, different mathematical models,
29:08 and push that to the device so it runs that on the well. So you have different degrees of complexity depending on how, you know, advanced the operator is. Do you work only with certain kinds of
29:20 lift or is it pretty much across the board? today. So.
29:25 there are two things, like the, let's say the, the ecosystem of devices and the data pipeline, that stuff is pretty agnostic. But I think what you want to do, I mean, what we believe in is what
29:36 you want to do is you don't want to serve people data, you really want to add the value out of processing and telling them like this is what's going on and this is a problem and hear that fix it or
29:46 just simply driving the wells. So there is that piece of component that's more application oriented part is very specific to case-by-case So today we started with, we started, initially we planned
29:57 to just be doing like basically pump jacks. And very quickly talking with operators, we realized, well, the pump jack's not leaving on its own, it has a tank battery. So we added the tank
30:05 battery. And by the way, it also has an injection well that's connected to it. So we also do the injection well. So today basically we do primarily pump jack, all the facilities around them. So
30:13 tanks, injection, flometers, kind of stuff. And we've done a little bit of work on some small gas lift systems, But we are building a lot of integration. or basically, well, that already have
30:27 some controllers where the data is stranded. So being able to integrate that with that, ESPs, total flow. So that's like a pretty standard device to measure gas flows. Am I forgetting anything
30:40 short term? No, we've kind of put the gas market aside. Frankly, we were talking about plunger lifts for a while. Reality's price of gas hasn't been that high Pretty much since we started the
30:51 company. So in general, there's, you know, this is why it doesn't make as much sense. So, so we focus on oil, but what you really want to do to Charles Point is you want to own a vertical and
31:04 no, you need to know the physics of every machine. If you just build a beautiful data system that just provides a lot of data, effectively, you just told the operator, you need to hire more
31:13 people to deal with this additional information I'm giving you, which most likely not going to do, right? people are already short staffed enough, and it's hard enough to find. engineers to do
31:25 the work. People have more and more wells per person, both at the field level, for the guys on the ground and at the engineering level. So you want to build the physics of every machine, you want
31:35 to build the right control, and then you roll that application out. But that's the relatively, you know, quantum code, the easy part. If you had everything, all the data, you observe
31:45 everything that happens, you know, you talk to all the industry, expert in the industry, and you can build something on top of it. It's, you know, building an app on the phone, right?
31:56 Simplifying things a little bit. But that's the system. And to go back to question, is it working? So we're now in 10 different states. So when we were here three years ago, we started in
32:09 Louisiana. We had to deal initially with an operator, which was very simple. We can do whatever we want on their field, as long as we break nothing. In exchange, that'll get a sweetheart price
32:20 when we're commercial.
32:28 And so, we spent a lot of time in that swamp. We expected me, I wouldn't have picked swampy Louisiana, but we spent our time in like horrible, humid weather in the swamp, like we're literally
32:40 back and forth, we're doing one week in Boston, one week in New Zealand, it's great very quickly on the hardware and you go from something that breaks every couple of months to something that lasts
32:48 10 years And Louisiana was actually perfect for this because it's hot, it's wet, it's the worst environment for electronic. We had all kinds of fun failures, like it turns out that deers like to
33:00 chew certain plastic. And so initially our old cable were polyurethane, I think it was, and it has a certain smell and the deers would come and chew those cables for the sensors. So we changed
33:13 that. We observed behavior like hogs have learned how to open some tank valves, like why did tank just drain itself at night. So they would create a little swamp so they can go, you know, like,
33:24 in a mud bath, in an old bath, basically, we saw everything over there. And it was the best place to build a system. And that operator had, you know, 600 something wells in the water flood. Uh,
33:35 so we grew from there. And now we're have, uh, between 1500 and thousand a system install out there. Yeah. Uh, on that, you just said water flood. So what, what, when we threw this, are you
33:46 guys, I'm assuming you're capturing information on, whether it's could be maybe a couple of different floods, right? You got water, flood, you got CO2 floods, steam floods, depending on what
33:55 you're at. You guys pulling in that data to tie into the optimization as well. Not yet. Not yet. Don't do optimization at the field level. Okay. That's the next, that was the vision when we
34:05 started. Yeah. And then we realized that it didn't match the reality of the field. It was like, okay, we don't optimize the whole field is going to be great. This is a smart field in a way.
34:14 Every single well is an optimizer. And the way to optimize a well, what do you need? I don't even have the data. You gotta call it before you run. Exactly, it was exactly that. So it's,
34:25 you know, I see it as this, I forgot, it's called this basic needs pyramid, right? For human, you need first food and water, then a shelter, then at the end is, you know, books and for your
34:34 brain. It's a little bit the same thing here. Everyone wants to fancy high-end application. That's the top of the pyramid. But if you don't have all the layers below, there's no point in even
34:43 starting there And there is also a sales side to this, which is we're a young company, and hopefully we come up as trustworthy people. But at the end of the day, we're a young company. People
34:55 don't know that's why we did trust us. And so if you are talking about effectively what you're describing is, you want to be optimizing the reservoir side of this thing. Now, immediately, the
35:04 timescales are months. And that means that you're a cell cycle because people are going to want to pilot it. the proof is in the pudding at the end of the day. So see what your results are. So now
35:14 you're talking months. Well, if you start with a more day to day operation side of things, like that's really quick. Like you, you get like you have a guy on location, Dean sells about 30
35:27 minutes within 30 minutes, you have data coming into the cloud. We run a couple of days of baseline. After that, we kick in, boom, one week later, your production is up 30 on this well, or
35:37 your runtime is down 70 on that well, and your production is not much. So all this kind of stuff, it's, it's really like concrete and really quick to show the value. So when you are a young
35:49 company, like having to prove yourself, that matters a lot to get that adoption where people can see quickly, okay, I tried, I see the benefit, okay, let's scale it up. Yeah. Well, once
35:59 you're on the reservoir side, immediately, and you know, reservoir engineers are,
36:05 it takes a lot to, I used to do reservoir work. So, It takes a lot to convince them, because there's a lot of fudge factor down there, lots of uncertainties, what's exactly is going on your
36:15 system. So immediately you are looking at timescales for a young business that are way, way longer. Yeah. On the software side, you pull the state in, what is the, there's a lot of analytics
36:27 out there, what's the differentiator, what makes you guys stand out? I think at the end of the day, it's
36:35 the, so it's a two thing One is the resolution and the level of detail. So, for instance, typically today, you have your S-Box system or whatever it is that you're, so talking about Rod Liff,
36:48 like Palm Jacks, one of the things you're gonna want to look at was called Dyna Card. So it's effectively like a little picture that tells you most of what you need to know about the health state of
36:57 the pump. It's a little bit simplistic, like there is value in bringing other sensors, but it's a good starting point the typically for most wealthy day people have.
37:10 a couple of those snapshots a day. Now, I mean, you know, you give up for you to well, your well starts, your pump may be full, at some point is gonna be empty, depending on when that snapshot,
37:21 like fail, you get a completely different story about what's going on. And then that's not, let's not talking about, sometimes you just have wells that aren't dynamic where you have gas, turf
37:30 yarns, slugs, things like that. So being able to systematically get for every single stroke of your well, get
37:40 the exact picture of, for every single stroke, here's what's going on. And like having that in context and being able to see exactly, to have this entire thing where you can tell exactly, okay,
37:53 yeah, we see what's going on, the loads we're here, now they're down there, okay. And at the same time, the pressure drop, okay, we just have a tubing leak, things like that. So that's one
38:03 part And then the second part is really looking at it like field-wired. as Sebastian weren't mentioning where. Now,
38:12 we don't do the full reservoir optimization, but the well is one part of the problem, and you have your well, but you also have the tanks, and so now you can crossfeed those data, you have your
38:23 injector, same thing. All of those things get crossfed so that you have, you can tell things, like for instance, there are wells where you're gonna have trade-offs between how hard you want to
38:34 push your equipment and how much production you want to make And sometimes, like sometimes it's clear cut, there's no question sometimes that just is a trade-off. So knowing what that's trade-off
38:44 and very often, like people have to rely basically on rule of thumbs on, how do I have to minimize that? It's like, ah, in this engineering group, like we want to push our well down to 70
38:53 village. This other group, it's 80. And all of that stuff, people just don't have the information to make like an informed decision. So it's kind of just, that's a wisdom, we've run by that,
39:04 kind of works for us, it's what it is So the idea is also to be able to. By crossing like that, pump level data with the production data, for instance, on this kind of problem, you can get much
39:14 more granular when you know exactly, okay, what is my, do I have a trade-off here, and if yes, where is it, and then it's up to the producer to say, well, on this one, I want to be pretty
39:25 conservative or more aggressive because more or less oil, more or less damage, though, so all things like that, bring you every piece of the puzzle together. And for the, that's also pretty key
39:35 for this peel side So obviously, as Seth mentioned, like, methane is becoming a big thing, but liquids feel like being a big problem for a long time and you drop saltwater on the ground, like,
39:49 nothing is going to grow there for the next 50 years, right? So you don't want that at the end of the day, like, people want to have good relations with neighbors, often they're like cattle,
40:00 farmland around, want to be respectful of those people being able to, again, being able to know, okay. what exactly is going on, which of my words are running, what performance are they rolling
40:11 at, how much liquid am I making? Uh oh, everyone is pumping, I don't have downtime, but I'm making half of what I should be making, I have a problem somewhere. Yeah. Where do you guys see
40:23 yourself in five years?
40:27 So, don't overthink it, just tell me, pie in the sky, what's the vision? We want to keep growing the company. This is not a quick flip scheme We COVID put a little hand, it'll stop on our
40:38 international ambition. This is all coming back now. We're starting to do our first deployment overseas.
40:44 Conclusion for us is clear. There's a huge need for what we do.
40:50 Basically, today we get all our customers by word of mouth. We get a customer A, and then they intro us to their buddy, you know, BNC. Until the day, and then this podcast is gonna go out.
40:59 Exactly. Here we go. I trust you
41:04 And basically that works.
41:06 There's no limit to what you can do with the data. You can collect, right? There are limits of what we're capable of doing, but other people can build application on it, and that can be used.
41:15 Other very kind of similar industries can use similar process. So right now, the plan is to keep growing in this industry, in the US. There's a lot of potential, obviously, and then doing the
41:26 same thing overseas. Five years from now, we'll probably be still doing pretty much that, and we'll reassess at that time That's five years in the startup life is a long time. It is, it's an
41:41 eternity. We've only been doing this for six years total, so it's like, so much can change so many different times. You just, you never really know what the futures hold, but keep listening to
41:51 your customers, and you just keep your, keep on it. You'd be surprised. Price was here where you go, and it's been really cool to see, like you said, you guys were here three years ago. When
42:01 you first kicked it off, you guys are just in Louisiana, you hear back growing. So it's so cool to see y'all's journey and we'll have you guys on again soon and continue to fall on. Looking
42:12 forward to that. Pleasure being here. Absolutely, guys. Hey y'all, take two seconds. We've already reviewed your shares with your friends. We'll catch you guys in the next episode.
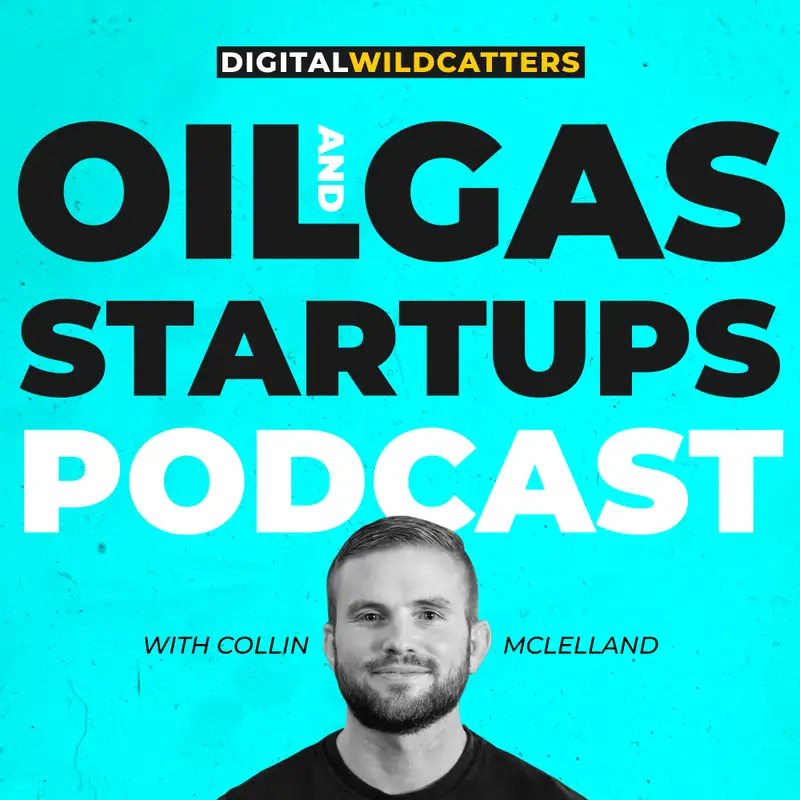